Astromining: detecting weak time-varying signals in large surveys using artificial intelligence.
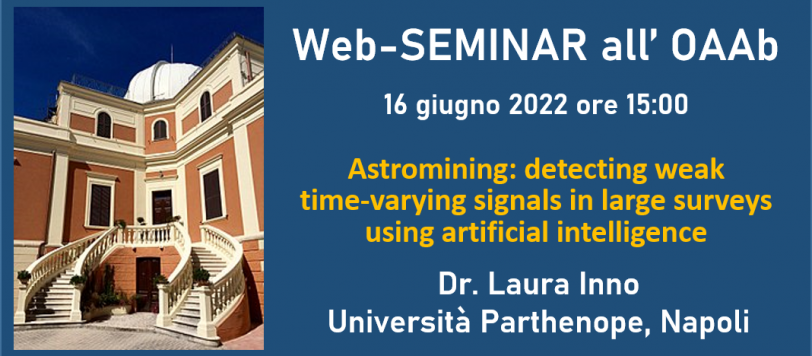
Giovedì 16 giugno alle ore 15:00, la Dr. Laura Inno dell’Università Parthenope di Napoli terrà il web-seminar dal titolo: The Vera C. Rubin LSST role in support of Comet Interceptor, the ESA F-class mission to an ancient word.
Abstract
Observational astronomy has now fully entered the Big Data domain: large photometric and spectroscopic surveys, either space or ground-based, are flooding the field with an overwhelming quantity of high-dimensional data. In this context, resorting to machine-learning methodologies is a natural choice to efficiently harvest the scientific content hidden in the data.
I will discuss two case studies that present high challenges and are related to planetary science. The first concerns the identification of exoplanets with the transit technique in the the space-born NASA mission, TESS. TESS produced over 1,000,000 light curves per month during the past 4years and it is still observing. Yet, planet candidates vetting from TESS data still rely on human efforts. This approach is obviously time consuming and it significantly weights down TESS potential for exoplanets’ discovery. In order to offer an automated alternative, we are building a neural network to perform vetting of planet candidates. Preliminary results show that our model achieves better accuracy when compared to similar existing software. The second case study concerns the detection of distant Solar System objects in images taken by the Vera C. Rubin Observatory LSST survey. By surveying the entire Southern sky every three nights for ten years in multiple bands, LSST will produce an unprecedented catalog of celestial objects. The survey comes with a built-in automated pipeline working on differential imaging. But objects that do not show significant motion in consecutive visits will elude this pipeline. I will present the ongoing work for building a deep-learning algorithm -to be merged within the pipeline- able to spot such slow-moving objects in LSST multiple acquisitions.
Comments are closed.